Why have language models become so impressive? Many people say that it’s the size of the models. The large in ‘large language models’ has been thought to be key to the models’ success: as the number of parameters in a model increases, its performance increases.
Size Matters, But Why?
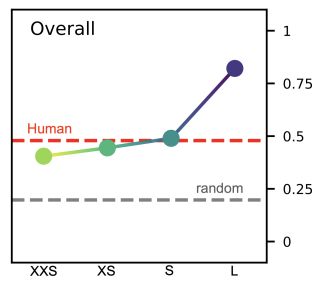
A selection of Figure 2 from Eisape et al.
Source: Tiwalayo Eisape / arXiv
There’s plenty of evidence that size matters when it comes to the performance of language models. For example, Tiwalayo Eisape and colleagues (2023) found that as the size of PaLM 2 increased from XXS to L, its performance on a logical task increased, eventually surpassing human performance (Figure 2).
But the more interesting question is not whether size matters to the performance of language models, but why. Fortunately, Eisape and colleagues addressed this question as well. They tested which aspects explained the most variance in the model’s behavior. Most of the variance (77 percent) was explained by one principal component: whether the model reconsidered the conclusion and searched for counterevidence (PC1 in Figure 7).
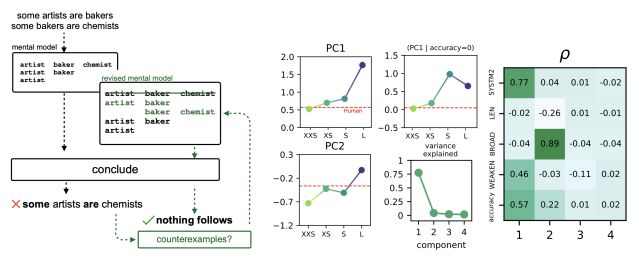
Figure 7 from Eisape et al. 2023
Source: Tiwalayo Eisape / arXiv
For more than a decade, philosophers and scientists have considered these two factors to be the core features of reflective or System 2 thinking (Kahneman 2011; Korsgaard 1996; Shea and Frith 2016; Byrd 2021, 2022). One way for machines to overcome faulty responses may be what seems to work for humans: step back from the initial impulse and consider more reasons (Belini-Leite 2023; Byrd and colleagues 2023).
Does Psychology Matter as Much as Size?
If the benefits of increasing the size of a language model are largely explained by the model’s engagement in reflective reasoning, then can smaller language models perform as well as larger models if we design the smaller models to think more reflectively?
Junbing Yan and colleagues (2023) compared the performance of multiple language models on two benchmarks. There were two key differences between the benchmarked language models: their size (the number of parameters) and their psychology (whether the model included only an intuitive system or also included a reflective system). Yan and colleagues’ Table 3 shows that psychology trumped size. Adding a reflective system to a small language model allowed it to outperform a language model that had 25 times more parameters. Even when that larger model was able to use more advanced prompt techniques, the smaller models that had a reflective system could keep up.
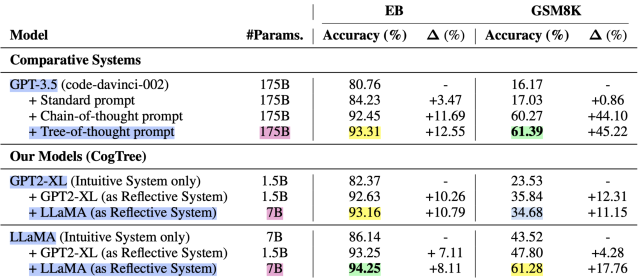
Table 3 from Yan and colleagues (highlighting added).
Source: Chengyu Wang / arXiv
Conclusion
These results not only confirm the diminishing returns on increasing the size of large language models. The results also suggest that before language models became as large as they are today, their size may have mattered less than their psychology. This means that AI companies may need to compete on psychological architecture rather than size. For psychology, this serves as a reminder that our reasoning strategies may be at least as important as other cognitive factors like cognitive capacity (Thompson and Markovits 2021).