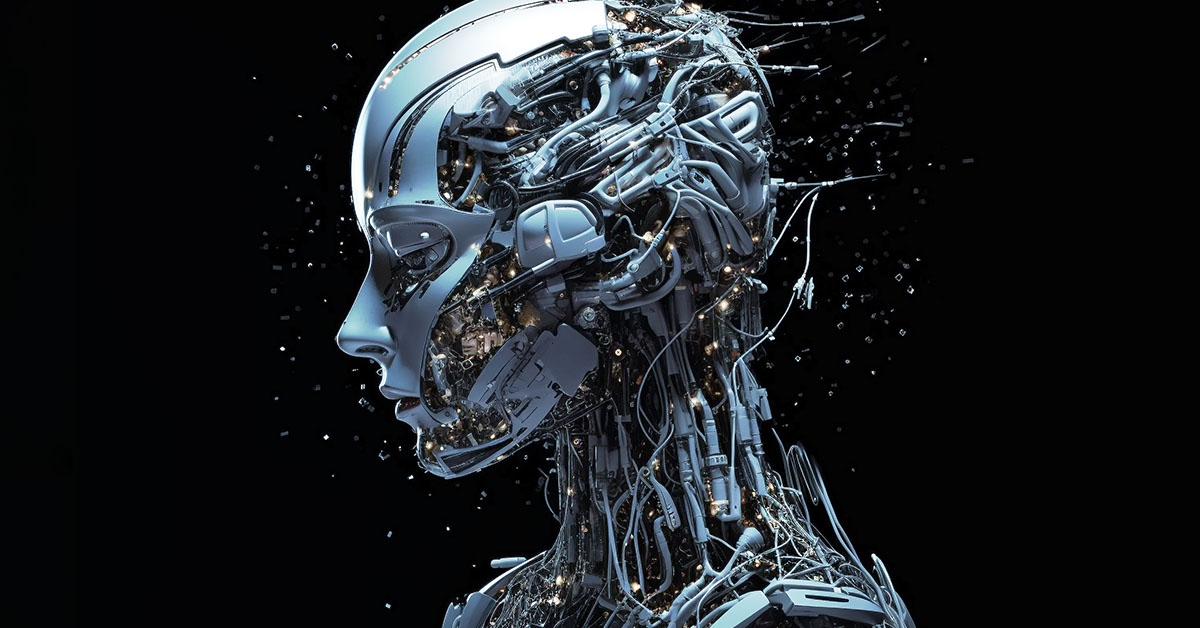
Artificial Intelligence (AI) is set to further improve in 2024, with large language models poised to advance even further.
2023 was an exciting year for AI with Generative AI, in particular those employing Large Language Model (LLM) architecture with the likes of the models from Open AI (GPT 4), Anthropic (Claude), and Open Source Community (Llama 2, Falcon, Mistral, Mixtral, and many more) gaining momentum and rapid adoption.
2024 may turn out to be an even more exciting year as AI takes centre stage everywhere including at CES 2024 and large language models are expected to advance even further.
What is Artificial Intelligence (AI) and What Stage Are We At?
AI deals with the area of developing computing systems that are capable of performing tasks that humans are very good at, for example recognising objects, recognising and making sense of speech, and decision making in a constrained environment.
Narrow AI (ANI): the field of AI where the machine is designed to perform a single task, and the machine gets very good at performing that particular task. However, once the machine is trained, it does not generalise to unseen domains. This is the form of AI, for example, Google Translate, represented the era of AI that we were in until recent times.
Broad AI (ABI): MIT IBM Watson Lab explain that “Broad AI is next. We’re just entering this frontier, but when it’s fully realized, it will feature AI systems that use and integrate multimodal data streams, learn more efficiently and flexibly, and traverse multiple tasks and domains. Broad AI will have powerful implications for business and society.”
IBM further explain that “Systems that execute specific tasks in a single domain are giving way to Broad AI that learns more generally and works across domains and problems. Foundation models, trained on large, unlabelled datasets and fine-tuned for an array of applications, are driving this shift.”
The emergence of Broad AI capabilities is recent with Francois Chollet (On the Measure of Intelligence) arguing in 2019 that “even the most advanced AI systems today do not belong to this (broad generalization) category…”
For more on the journey of Broad AI see Sepp Hochreiter, Toward a Broad AI (2022): “A broad AI is a sophisticated and adaptive system, which successfully performs any cognitive task by virtue of its sensory perception, previous experience, and learned skills.”
However, the author clarifies that ABI models do not possess the overall general capabilities of the human brain.
Artificial General Intelligence (AGI): a form of AI that can accomplish any intellectual task that a human being can do. It is more conscious and makes decisions similar to the way humans make decisions. It is also referred to as ‘strong AI’ and IBM describe AGI or Strong AI as possessing an intelligence equal to humans with self-aware consciousness and an ability for problem solving, learning and planning for the future. In effect it would result in ‘intelligent machines that are indistinguishable from the human mind.
AGI remains an aspiration at this moment in time, with various forecasts ranging from 2025 to 2049 or even never in terms of its arrival. It may arrive within the next decade, but it has challenges relating to hardware, the energy consumption required in today’s powerful machines. The author personally believes that the 2030s is a more probable arrival time.
Artificial Super Intelligence (ASI): is a form of intelligence that exceeds the performance of humans in all domains (as defined by Nick Bostrom). This refers to aspects like general wisdom, problem solving, and creativity. The author’s personal view is that humans will use human computer interfaces (possibly a wireless cap or headset) to leverage advanced AI and become the ASI (perhaps a merger between Neuromorphic Computing combined with Quantum capabilities in the future and is referred to as Quantum Neuromorphic Computing).
Where Are We in Terms of AI Today?
The arrival of GPT-4 from Open AI triggered a great deal of debate across social media with some commenting that as GPT-4 was not narrow AI it therefore had to be Artificial General Intelligence (AGI). The author will explain that the latter is not the case.
AGI is unlikely to magically appear overnight and rather it is more likely to arrive via a process of ongoing evolutionary advancement in AI research and development.
We have been in the era of Narrow AI until recently. However, many of the State-of-the-art (SOTA) models can now go beyond narrow AI (ANI) and increasingly we are experiencing Generative AI models utilising LLMs that are in turn applying Transformers with Self-Attention Mechanism architecture and they are able to demonstrate multimodal, multitasking capabilities.
Nevertheless, it would not be accurate to state that the current SOTA models are at the human brain level, or AGI, in particular on logic and reasoning tasks (including common sense).
We are in the era of broad AI (or Artificial Broad Intelligence, ABI) whereby the Generative AI models are neither narrow (Artificial Narrow Intelligence, or ANI) as they can perform more than one task, but neither AGI as they are not at the level of the intelligence and capabilities of the human brain.
The advanced robots of the sci-fi movies have not been present in our everyday lives, however as AI technology advances AI is increasingly being embedded into advanced robotics and the robot technology is rapidly advancing for example Stanford based researchers introduced Mobile Aloha robot that learns from humans to cook, clean and do the laundry.
Furthermore, Machine Learning engineer Santiago Valdarrama demonstrated how to incorporate ChatGPT into Boston Dynamics robot Spot.
The Pathway Towards Advanced AI Capabilities
-
Memory: it is rumoured that GPT-5 will address this issue and other models have been seeking to address the memory issue so that the AI agent recalls previous engagement. Furthermore in terms of memory and LLMs it is worth noting Dale Schuurmanns paper (2023) with an overview provided by Jesus Rodriguez. And the potential of Langchain. Wang et al. (2023) published Augmenting language Models with Long-Term Memory.
-
Logic, reasoning, causal inference: common sense and causal inference is an area where LLMs and other areas of Deep Neural Networks / Machine Learning models often struggle. Whilst Chain of Thought (CoT) has shown promise, Generative AI models are far short of advanced human logical reasoning. It may be that Neurosymbolic approaches including leveraging symbolic AI via plugins to LLMs will help address these issues in future and set a pathway towards AGI.
-
Learning from smaller data sets: zero shot learning and zero-shot prompting, with self-supervised learning employed by Transformers with Self-Attention Mechanism to advance the state of AI capabilities.
-
Continued, updated knowledge of the world beyond the initial training – RAG: Retrieval-Augmented Generation enables LLM models to connect to external data sources via the internet or private data via frameworks such as Langchain or LlamaIndex and retrieve up-to-date information.
-
Dynamic responses to an uncertain world / learning on the fly.
-
Mutlimodal multitasking: LLMs are developing multimodal, multitasking capabilities and GPT 5 from Open AI is expected to demonstrate these, as will other models.
-
Data: access, effective and efficient storage, security and quality are all key for AI models. Increasingly synthetic data, that may itself be created by Generative AI models may play a key role in AI going forwards
-
Model behaviour – Reinforcement Learning (RL): Reinforcement Learning from Human Feedback (RLHF) is applied to LLMs to reduce the bias whilst at the same time also increasing the performance, fairness, and representation. RLHF entails a dynamic environment whereby the AI agent is seeking to maximise rewards (received for the optimal or at least better actions) as it enters a new state (actions taken by the AI agent) with a good overview provided in What is RLHF. See also LLM Training: RLHF and Its Alternatives and Illustrating Reinforcement Learning from Human Feedback (RLHF) with the discussion about the potential for Implicit language Q Learning (ILQL) to take over in the future.
Even when all the above are fully accomplished, the author argues that we don’t have machine intelligence and capabilities to match a human brain due to the computational and resource efficiency challenge, in particular energy consumption.
Joey deVilla in “The best social media post about AGI that you might have missed” (Jan 2024) flagged the post on Threads by Buzz Andersen that sums up the challenge for AGI that genuinely matches the capabilities of the human brain:
ChatGPT (3.5) and GPT 4 from Open AI have been extremely impressive models in terms of capabilities. However, we need to address the issue of advancing performance and capabilities of models with energy efficiency,
ChatGPT is reported to consume as much energy as 33,000 US households on a daily basis and as may have consumed as much electricity as 175,000 Danes consumed in January 2023!
In an era of transitioning to a low carbon footprint such consumption we need to move towards greater computational resource efficiency. This is also desirable from an economics perspective as firms seek to scale AI on a cost efficient basis.
Furthermore, in time with the arrival of AGI some researchers warn of the dangers of AGI and energy consumption and in effect a competition with humans for energy supply.
For example, AI researchers have flagged the risks of advanced AI models competing with humans for energy and turning our planet into data centres and solar panels everywhere.
Moreover, Research Scientists at Google DeepMind and University of Oxford authored a paper that an advanced AI would compete with humans for limited energy supply.
However, the flip side as pointed out by the IEA is that AI may in fact play a key role in helping efficiently manage complex power grids.
Hence, the question is how should society manage the negative risks of powerful AI models vs the upside benefits. A starting point would be to make the models more resource efficient including from an energy consumption perspective (which in turn delivers economic cost benefits to companies and users too).
Techniques Being Employed to Make LLMs More Efficient (Non-Exhaustive List)
Both the tech majors and the Open-Source Community have been advancing approaches that make LLM models more efficient. For the Open-Source community, it is important to find solutions on efficiency as many in the community lack the resources of a large major. However, even the tech majors are increasingly aware that scaling massive LLM models to vast number of users results in huge server plus energy costs and as a result is not so good for carbon footprint.
Examples of advances in making Generative AI models more efficient:
LoRA (Low-Rank Adaptation of Large Language Models)
LoRA: Is a technique that results in a material reduction in the number of parameters during training and is achieved via the insertion into the model of a lesser number of new weights with only these new weights being trained. This in turn results in a training process that is significantly faster, memory efficient and easier to share and store due to the reduced model weights.
Flash Attention is another innovation that is being considered for fast and memory efficient exact attention with Input and Output Awareness.
Model pruning: These non-essential components can be pruned, making the model more compact while maintaining the model’s performance.
LLM Quantization: Quantization, a compression technique, converts these parameters into single-byte or smaller integers, significantly reducing the size of an LLM.
In addition, the hardware solutions may offer computational resource efficiencies that also result in energy and hence carbon footprint savings for example 5th Gen Intel Xeon Scalable Processor, the work that IBM are undertaking with an analog AI chip and others. This will enable the rise of the AIoT whereby AI scales across the edge of the network across devices in power constrained environments where efficiency and low latency is key.
Source for image above: Intel (stats 5th Gen Intel Xeon Processors vs 4th Gen).
Firms may wish to consider model architectures that balance performance capabilities alongside resource costs (computational costs including energy and carbon footprint) and hardware the net present value returns (NPV) or return on investment (ROI) that efficient hardware such as 5th Gen Intel Xeon Scalable Processors may deliver in particular for inferencing and /or fine tuning models with low latency in models with less than 20Bn parameters in size as proposed by the author previously.
The author believes in the longer-term that Quantum Computing may provide potential a pathway to advancing AI towards ASI, however, a possible pathway towards AGI (that is also energy efficient) may be provided by Spiking Neural Networks combined with Dendritic Computing, with Neuromorphic Computing.
Spiking Neural Networks (SNNs) in particular when combined with Dendritic Computing are more closely aligned to our own human brains relative to a typical Artificial Neural Network (ANN) architecture in Deep Learning. SNNs are more energy efficient relative to ANNs, and can be architected to be ultra-low latency, may engage in continuous learning and as they can be deployed on the edge of the network (referring here the device itself) they can be more data secure too.
Neuroscientists have found that Dendrites help explain our brain’s unique computing power and it was reported for the first time that scientists observed a form of cell messaging within the human brain that was considered unique and potentially indicates that our human brain possesses greater computational power than previously believed.
“The Dendrites are central to understanding the brain because they are at the core of what determines the computational power of single neurons,” neuroscientist (based at Humboldt University) Matthew Larkum stated to Walter Beckwith (Dendrite Activity May Boost Brain Processing Power) at the American Association for the Advancement of Science in January 2020.
Research has set out the potential computational advantages of dendritic amplification and the potential for leveraging dendritic properties to advance Machine Learning and neuro- inspired computing.
Moreover, research has also shown that the Dendrite alone can perform complex computations, and hence the multi-parallel processing power of a single neuron is far beyond what was conventionally assumed.
In addition, researchers are seeking to better understand how memory is stored in Dendritic spines within the brain and the potential to treat diseases such as Alzheimer’s. This points to Dendrites playing an important role in the human brain and yet ANN architectures don’t possess Dendrites (nor do a number of SNN architectures that have been emerging in recent years albeit the author believes this will change going forwards).
Furthermore, Dendrite pre-processing has been shown to reduce the size of network required for a threshold performance. Furthermore, SNNs with Dendritic Computing could entail running on watts instead of Megawatts.
By leveraging analog signals and continuous dynamics, neuromorphic computing can improve the speed, accuracy, and adaptability of AI applications while overcoming traditional computing’s limitations, such as latency, power consumption, and scalability.
This will lead to the Internet of Everything (IoE) where efficient AI agents will transcend locally across all internet connected devices providing intelligent responses and hence mass hyper-personalisation at scale across all interactions, in turn referred to as the AIoE.
The AIoT and then following on the AIoE is a world where devices communicate to each other and dynamically interact with humans.
Examples of Neuromorphic Computing include:
Moreover, Neuromorphic Computing may play an essential role in enabling mixed reality glasses given the powerful computational needs along with the energy constraints and need for low latency.
The author applauds the work of the likes of Jason Eshraghian et al. (2023) for their work on SpikeGPT and this article also serves as an invitation to Jason Eshraghian and other researchers to explore collaborations in this area.
The author believes that in time Neuromorphic Computing entailing Spiking Neural Networks (SNNs) with Dendritic Computing will enable the emergence of efficient, advanced AI that is closer to the human brain and human level intelligence. The author suggests that the realistic timeline for the emergence of ‘genuine AGI’ (that truly matches the capabilities of the human brain) is likely to occur in the 2030s. Foresight Bureau predicts the arrival of AGI in 2030 and Demis Hasibis of DeepMind stated that “I don’t see any reason why that progress is going to slow down. I think it may even accelerate. So I think we could be just a few years, maybe within a decade away.”
However, AI pioneer Herbert A. Simon had predicted that AGI would arrive by 1985 and hence it is worth noting that no one can really state with certainty when AGI will actually arrive with Geoffrey Hinton stating that “I now predict 5 to 20 years but without much confidence… Nobody really knows…”
This article is a strategic analysis of the current state of AI the author’s strategic vision of what may come next, however, it is not intended to be used on a reliance basis nor provide any representations or warranties implicitly or explicitly and for those seeking to venture into the field of AI and LLMs it is important to conduct their own due diligence and assessments as to which models, pipelines and technical hardware solutions are appropriate for their use case and situation as results may vary.
The next article in this series will consider how we may lay the foundations for the successful emergence of AGI and indeed for scaling ABI in terms of ethics and ensuring ESG values (including data governance).
About the Author
Imtiaz Adam is a Hybrid Strategist and Data Scientist. He is focussed on the latest developments in artificial intelligence and machine learning techniques with a particular focus on deep learning. Imtiaz holds an MSc in Computer Science with research in AI (Distinction) University of London, MBA (Distinction), Sloan in Strategy Fellow London Business School, MSc Finance with Quantitative Econometric Modelling (Distinction) at Cass Business School. He is the Founder of Deep Learn Strategies Limited, and served as Director & Global Head of a business he founded at Morgan Stanley in Climate Finance & ESG Strategic Advisory. He has a strong expertise in enterprise sales & marketing, data science, and corporate & business strategist.