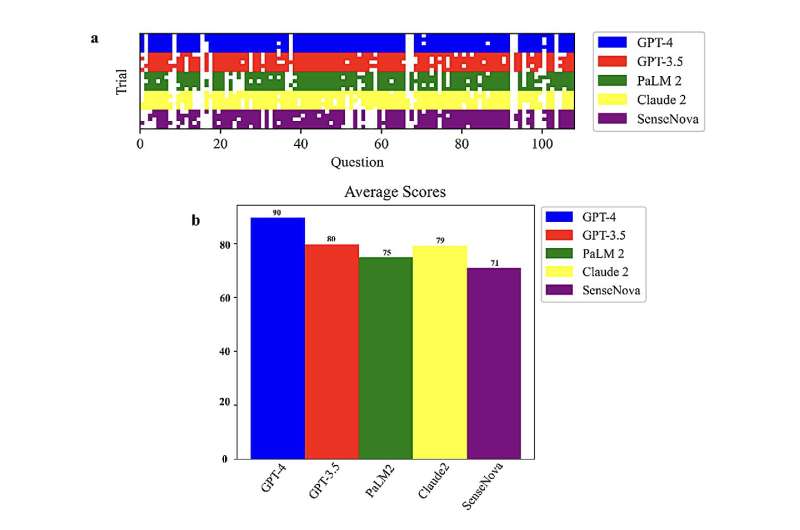
Large language models (LLMs) are advanced deep learning algorithms that can process written or spoken prompts and generate texts in response to these prompts. These models have recently become increasingly popular and are now helping many users to create summaries of long documents, gain inspiration for brand names, find quick answers to simple queries, and generate various other types of texts.
Researchers at the University of Georgia and Mayo Clinic recently set out to assess the biological knowledge and reasoning skills of different LLMs. Their paper, pre-published on the arXiv server, suggests that OpenAI’s model GPT-4 performs better than the other predominant LLMs on the market on reasoning biology problems.
“Our recent publication is a testament to the significant impact of AI on biological research,” Zhengliang Liu, co-author of the recent paper, told Tech Xplore. “This study was born out of the rapid adoption and evolution of LLMs, especially following the notable introduction of ChatGPT in November 2022. These advancements, perceived as critical steps towards Artificial General Intelligence (AGI), marked a shift from traditional biotechnological approaches to an AI-focused methodology in the realm of biology.”
In their recent study, Liu and his colleagues set out to better understand the potential value of LLMs as tools for conducting research in biology. While many past studies emphasized the utility of these models in a wide range of domains, their ability to reason about biological data and concepts has not yet been evaluated in depth.
“The primary objectives of this paper were to assess and compare the capabilities of leading LLMs, such as GPT-4, GPT-3.5, PaLM2, Claude2, and SenseNova, in their ability to comprehend and reason through biology-related questions,” Liu said. “This was meticulously evaluated using a 108-question multiple-choice exam, covering diverse areas like molecular biology, biological techniques, metabolic engineering, and synthetic biology.”
Liu and his colleagues planned to determine how some of the most renowned LLMs available today process and analyze biological information, while also assessing their ability to generate relevant biological hypotheses and tackle biology-related logical reasoning tasks. The researchers compared the performance of five different LLMs using multiple-choice tests.
“Multiple-choice tests are commonly used for evaluating LLMs because the test results can be easily graded/evaluated/compared,” Jason Holmes, co-author of the paper explained. “For this study, biology experts designed a 108-question multiple-choice test with a few subcategories.”
Holmes and their colleagues asked LLMs each of the questions in the test they compiled five times. Every time a question was asked, however, they changed how it was phrased.
“The purpose of asking the same question multiple times for each LLM was to determine both the average performance and the average variation in answers,” Holmes explained. “We varied the phrasing so as not to accidentally base our results on an optimal or suboptimal phrasing of instructions that led to a change in performance. This approach also gives us an idea of how the performance will vary in real world usage, where users will not be asking questions in the same way.”
The tests carried out by Liu, Holmes and their colleagues gathered insight on the potential utility of different LLMs for assisting biology researchers. Overall, their results suggest that LLMs respond well to various biology-related questions, while also accurately relating concepts rooted in fundamental molecular biology, common molecular biology, metabolic engineering and synthetic biology.
“Notably, GPT-4 demonstrated superior performance among the examined LLMs, achieving an average score of 90 on our multiple-choice tests across five trials utilizing distinct prompts,” Xinyu Gong, co-author of the paper, said.
“Beyond attaining the highest test score overall, GPT-4 also exhibited great consistency across the trials, highlighting its reliability in biology reasoning compared to peer models. These findings emphasize GPT-4’s immense capacity to assist biology research and education.”
The recent study by this team of researchers could soon inspire additional work that further explores the usability of LLMs in the field of biology. The results gathered so far suggest that LLMs could be useful tools for both research and education, for instance supporting the tutoring of students on biology, the creation of interactive learning tools and the creation of testable biological hypotheses.
“In essence, our paper represents a pioneering effort in merging the capabilities of advanced AI, particularly LLMs, with the intricate and fast-evolving field of biology,” Liu said. “It marks a new chapter in biological research, positioning AI not just as a supportive tool, but as a central element in navigating and deciphering the vast and complex biological landscape.”
The future advancement of LLMs and their further training on biological data could pave the way for important scientific discoveries, while also enabling the creation of more advanced educational tools. Liu, Holmes, Gong and their colleagues are now planning to conduct further studies in this area.
In their next works, they first plan to devise strategies to overcome the computational demands and privacy-related issues associated with the use of GPT-4, the LLM underpinning ChatGPT. This could be achieved by developing open-source LLMs to automate tasks such as gene annotation and phenotype-genotype pairing.
“We’ll employ knowledge distillation from GPT-4, creating instruction-following data to fine-tune local models such as the LLaMA foundation models,” Zihao Wu, co-author of the paper, told Tech Xplore.
“This strategy will leverage GPT-4’s capabilities while addressing privacy and cost concerns, making advanced tools more accessible to the biology community. Additionally, with GPT-4V’s vision capabilities, we’ll extend our research to multimodal analyses, focusing on natural drug molecules, such as anti-cancer agents or vaccine adjuvants, particularly those with unknown biosynthetic pathways.”
“We’ll investigate their chemical and biosynthetic pathways and potential applications. GPT-4V’s ability to recognize molecular structures will enhance our analysis of complex multimodal data, advancing our understanding and application in drug discovery and development in synthetic biology.”
More information:
Xinyu Gong et al, Evaluating the Potential of Leading Large Language Models in Reasoning Biology Questions, arXiv (2023). DOI: 10.48550/arxiv.2311.07582
© 2023 Science X Network
Citation:
Testing the biological reasoning capabilities of large language models (2023, December 19)
retrieved 22 December 2023
from https://techxplore.com/news/2023-12-biological-capabilities-large-language.html
This document is subject to copyright. Apart from any fair dealing for the purpose of private study or research, no
part may be reproduced without the written permission. The content is provided for information purposes only.