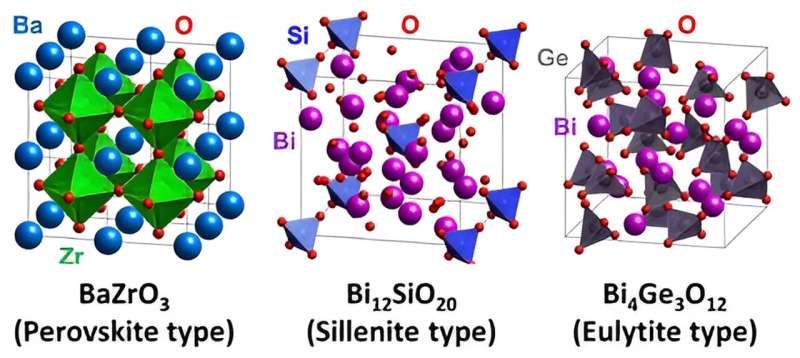
Researchers at Kyushu University, in collaboration with Osaka University and the Fine Ceramics Center, have developed a framework that uses machine learning to speed up the discovery of materials for green energy technology.
Using the new approach, the researchers identified and successfully synthesized two new candidate materials for use in solid oxide fuel cells—devices that can generate energy using fuels like hydrogen, which don’t emit carbon dioxide.
Their findings, which were reported in the journal, Advanced Energy Materials, could also be used to accelerate the search for other innovative materials beyond the energy sector.
In response to a warming climate, researchers have been developing new ways to generate energy without using fossil fuels.
“One path to carbon neutrality is by creating a hydrogen society. However, as well as optimizing how hydrogen is made, stored and transported, we also need to boost the power-generating efficiency of hydrogen fuel cells,” explains Professor Yoshihiro Yamazaki, of Kyushu University’s Department of Materials Science and Technology, Platform of Inter-/Transdisciplinary Energy Research (Q-PIT).
To generate an electric current, solid oxide fuel cells need to be able to efficiently conduct hydrogen ions (or protons) through a solid material, known as an electrolyte. Currently, research into new electrolyte materials has focused on oxides with very specific crystal arrangements of atoms, known as a perovskite structure.
“The first proton-conducting oxide discovered was in a perovskite structure, and new high-performing perovskites are continually being reported,” says Professor Yamazaki. “But we want to expand the discovery of solid electrolytes to non-perovskite oxides, which also have the capability of conducting protons very efficiently.”
However, discovering proton-conducting materials with alternative crystal structures via traditional “trial and error” methods has numerous limitations.
For an electrolyte to gain the ability to conduct protons, small traces of another substance, known as a dopant, must be added to the base material. But with many promising base and dopant candidates—each with different atomic and electronic properties—finding the optimal combination that enhances proton conductivity becomes difficult and time-consuming.
Instead, the researchers calculated the properties of different oxides and dopants. They then used machine learning to analyze the data, identify the factors that impact the proton conductivity of a material, and predict potential combinations.
Guided by these factors, the researchers then synthesized two promising materials, each with unique crystal structures, and assessed how well they conducted protons. Remarkably, both materials demonstrated proton conductivity in just a single experiment.
One of the materials, the researchers highlighted, is the first-known proton conductor with a sillenite crystal structure. The other, which has a eulytite structure, has a high-speed proton conduction path that is distinct from the conduction paths seen in perovskites.
Currently, the performance of these oxides as electrolytes is low, but with further exploration, the research team believes their conductivity can be improved.
“Our framework has the potential to greatly expand the search space for proton-conducting oxides, and therefore significantly accelerate advancements in solid oxide fuel cells. It’s a promising step forward to realizing a hydrogen society,” concludes Professor Yamazaki.
“With minor modifications, this framework could also be adapted to other fields of materials science, and potentially accelerate the development of many innovative materials.”
More information:
Susumu Fujii et al, Discovery of Unconventional Proton‐Conducting Inorganic Solids via Defect‐Chemistry‐Trained, Interpretable Machine Learning, Advanced Energy Materials (2023). DOI: 10.1002/aenm.202301892
Citation:
Machine learning method speeds up discovery of green energy materials (2024, January 18)
retrieved 18 January 2024
from https://techxplore.com/news/2024-01-machine-method-discovery-green-energy.html
This document is subject to copyright. Apart from any fair dealing for the purpose of private study or research, no
part may be reproduced without the written permission. The content is provided for information purposes only.