What happens when you finish ChatGPT and DALL-E? OpenAI has set out to keep the whole tech industry guessing without wasting a moment. Although its IT sector rivals could not match OpenAI’s inventive zeal, the latter firmly established itself as the unrivaled frontrunner in artificial intelligence research. The increasing homogeneity of modern technology begs the question: what are the defining features of these cutting-edge tools?
Generative AI, which can produce new material from basic ideas, has been largely responsible for AI’s impressive advancements. This includes AI-generated graphics, text, and audio files. Today 92% of companies are using ChatGPT for their business operations which shows the future growth for AI.
Generative AI, thankfully, isn’t as complex as it appears; the idea is to train a model to look for similarities in incoming data and then generate new data that is comparable to the input. The next question is, assuming that these models improve and learn from their errors with ongoing training, what more can they do? This article will cover a complete guide to Generative AI models.
Generate AI History and Development: A Brief Overview
Beyond amusing smartphone applications and avatars, AI is also being used to generate high-quality artwork, design, coding, blog entries, and more. Generative AI uses unsupervised and semi-supervised learning to process huge amounts of data and develop its results. Big language models boost computers’ understanding and creation of creative material. The neural network behind generative AI may learn from pictures or words and change its properties.
Thanks to generative AI Development, computers can anticipate the most essential patterns by generating fake human input. Generative AI models may form conclusions and highlight particular training data with few training parameters. However, successful generative AI training requires human participation before and after exercise.
Must Read: The Role Of Generative AI In Architecture
Possible generational AI usage
Many creative businesses employ generative AI to create visual art and other items. Artificial intelligence graphics are becoming art. These models may be trained on many paintings, resulting in works with similar subjects but different styles.
To teach your AI Leonardo da Vinci’s style, show it several of his works. After feeding the artwork into the model’s neural network, it creates carbon duplicates. Models generate text, audio, etc. By changing style or concentrating on sections of a picture, generative AI may create new ones. This occurs when a generative AI model tries to copy your favorite art. A refined design based on early sketches or wireframes may be offered.
Generative AI revolutionizes video game development by creating new levels, chat options, maps, and virtual environments. Generative AI might transform gaming by developing new planets, towns, woodlands, and other immersive settings. The scenario shows how to train generators to replicate game visual output. Generative AI creates new video game genres and makes more games every year.
-
Health Care Professionals
Generative AI models may help physicians detect and cure health issues quickly. MRI to CT scan conversion is a medical usage of generative AI. Generative adversarial networks may train and build models using fake data. They also excel at data identification, privacy, and security.
Generative AI can interpret audio data and modify music and speech pitches. Its application in music may blend rock and classical styles. An AI-powered software engine called Musico can make music from codes, actions, gestures, and more. The user may create a musical sketch or a whole song using Musico’s engines.
Web and email marketers need more automation to match the need for personalized content. Generative AI looks ideal for literature and copywriting. Copywriters can now educate generative AI systems to see the broad picture and locate appropriate terms to produce captivating headlines. Generative AI’s content-generation talents may help you improve your work, clarify your thoughts, and develop great material.
Generative AI systems help authors fulfill their creative potential, contrary to worries that they are invading copywriting and related professions. Copy.ai, a generative AI, can write compelling emails, social media messages, and blog articles without human input. Since computers can automate bullet points and subheadings, copywriters may concentrate on more creative tasks.
Generative AI and super-resolution might transform content production. Using machine learning in marketing may help media businesses create better content. Predictive targeting uses AI and ML to forecast client behavior based on historical data and trends.
Synthesia, like other notable generative AI businesses, employs cutting-edge synthetic media technology to let anybody create stunning visuals regardless of talent or budget. Generative AI might improve advertising and marketing by creating more relevant and exciting content, analyzing consumer behavior and the market, and creating more successful campaigns.
How generative AI is governed?
Different AI regulatory viewpoints have evolved with generative AI. Companies self-govern by managing release tactics, monitoring model usage, and restricting commodity access. This is one of two primary business methods. Other theories suggest that generative AI models help everyone get resources, benefiting businesses and society.
Government agencies’ unregulated use of generative AI models presents copyright, privacy, and intellectual property concerns. Plagiarism occurs when online generative AI datasets are used without permission. The dataset seems unique, yet it is manufactured.
Read more: The Evolution Of AI Assistants: Google Bard V/S ChatGPT
Problems with generative AI
Although beneficial, generative AI raises ethical, legal, and moral problems. Start by assessing the most pressing issues:
The unlimited manufacturing potential of generative AI will flood the internet with copies of artwork entirely different from the originals. Many creatives fear technology will replace human work. This comprises freelancers, commercial artists, and advertising, publishing, and entertainment professionals.
Generative AI models are trained on massive bias-prone datasets. Therefore, sifting reputable material from books, websites, and papers is hard. Because of this, models may create deep fakes, sustain ML bias, and propagate disinformation across networks.
Unfortunately, many dishonest persons and identity thieves may utilize generative AI to harm people or spread bogus news.
Key Types of Generative AI Models
-
Generative Adversarial Networks (GANs)
Generative Adversarial Networks (GANs), first discovered in 2014, were the most popular technique before the present success of diffusion models. In a GAN, two neural networks engage in a competitive process; one network learns to create new instances, while the other learns to distinguish between domain-based and generated information.
-
Variational Autoencoders (VAEs)
A VAE consists of two neural networks, one for encoding and one for decoding. Encoders take in data as input and compress it to a denser, more miniature representation while retaining crucial elements and discarding the rest. After decompressing the data, the decoder may return it to its original form.
When they work together to provide an efficient and straightforward latent data representation, it becomes easy to choose new latent representations that might give unique data. Compared to diffusion models, VAEs provide lower-quality images but can produce results faster.
-
Transformer-based Models (GPT-3, ChatGPT)
Models based on transformers may understand and generate language that sounds natural, for instance, OpenAI’s GPT-3 and ChatGPT. These models have captured the generative AI scene and found applications in various fields, from essay writing to conversational AI. Google Bard and ChatGPT are notable examples of this capacity that may produce complicated responses to user questions.
Read more: Super AI: Understanding The Definition, Threats, and Trends
Real-World Applications of Generative AI
-
Promotional Content and Writing
Generative AI development services have revolutionized the way ads and content are created. Models like this allow businesses to maintain a consistent online presence and improve audience communication since they produce high-quality, unique content at a considerably quicker pace than a human writer could. The Generative AI market size is expected to grow at a rate of 24.40%, resulting in a market volume of US$207.00 billion by 2030.
With the ability to generate ad copy, articles, blog entries, and social media updates, generative AI has become a powerful tool for marketers.
-
Personalized Customer Experience
Businesses may now deliver customers highly personalized experiences thanks to generative AI. Real-time conversational AI chatbots like ChatGPT and Google Bard may answer inquiries, provide ideas, and even handle customer complaints. They may be set up to sound like the company’s voice, so they will always have the same experience no matter what channel consumers use.
-
Evaluations of Target Audiences and Their Perspectives
Businesses may also benefit from generative AI’s ability to highlight their ideal customer profile. These models can process large amounts of data and identify patterns and trends, which could help with marketing, product development, and other company decisions. For instance, generative AI might analyze client sentiment towards a particular brand or product by filtering through social media posts and providing relevant input to the company.
-
Supplemental Information on Product Surveys and Details
Creating engaging and compelling product descriptions could be a huge time-sink for online stores. Generic artificial intelligence (AI) automation has the potential to generate unique, persuasive product descriptions that highlight the product’s strongest features. Similarly, these algorithms may generate surveys tailored to each respondent’s preferences and interests, which boosts response rates and data quality.
Assessing the Efficiency of Generative AI
-
Guaranteeing Reliable Outcomes
A critical aspect of generative AI is its ability to generate precise and accurate outcomes. The power of these models to learn and adapt over time might lead to results more convincingly attributed to human creators. The quantity and quality of the training data could affect their performance. The fact that these algorithms may provide seemingly trustworthy data that is incorrect emphasizes the need for human oversight.
-
The Efficiency and Quickness of Generative Models
Generative AI models excel in being both quick and adaptable. Generative AI models have a reputation for being unfeeling and sluggish. Their speed may be much lower than other AI models, such as classification or regression.
Generative models such as variational autoencoders (VAEs) and generative adversarial networks (GANs) need this kind of input for training. This procedure may be computationally intensive and time-consuming when creating complex, high-quality outputs like graphics or text. The time it takes for generative models to process inputs and generate suitable outputs can make them less responsive than other AI models.
-
Assessments of Performance and Practical Uses
Generative AI has become quite popular due to its versatility. For instance, artificial intelligence models might completely alter the entertainment industry by writing scripts, designing environments for video games, and even composing music. Generative AI allows educators to tailor classes to students’ unique needs, interests, and learning styles. Healthcare practitioners may also benefit from generative AI systems, which can analyze medical images and create synthetic data for research purposes.
Access and Cost of Generative AI Platforms
-
Analyzing Common Pricing Models
Generative AI system prices depend on model complexity, data volume, and use case. Companies that charge by the number of AI system inquiries may provide a pay-as-you-go alternative. Specific membership levels may have costs. Customers may try the tool’s free tiers from several providers before buying a premium subscription.
-
Comparing Generative AI to Traditional Methods Cost-wise
Comparing generative AI solutions to traditional methods should consider time and money savings. This is extra to the original investment. An AI model may be expensive initially, but it will save money by removing tedious, repetitive jobs. Since it is efficient and accurate, generative AI may provide superior outcomes at lower cost.
-
Generative AI ROI evaluation
The ROI of an AI generative solution may be measured by time saved, productivity enhanced, customer happiness, and future income creation. Different firms define “return on investment” (ROI) differently. A company may gain traffic, leads, or revenue using a generative AI system to produce content. Businesses may better understand generative AI technology’s worth if their benefits can be assessed.
The Future of Generative AI Looks Bright
In a world where most AI systems are limited to classifying data, generative AI stands out due to its innovative and creative thinking. Many experts are hopeful that new solutions will be developed in the coming years because of the increasing use of generative AI tools in industries like biotech, pharmaceuticals, and medtech. These devices are reshaping the arts, marketing, and domains. Most people think of OpenAI’s ChatGPT when they hear “generative AI.” The chatbot quickly gained millions of users after becoming viral. The scope of generative AI, however, goes beyond natural language processing (NLP) activities like translation, summarization, and text synthesis. The potential for generative AI to revolutionize internet use is huge, even if it is still in its early stages.
Conclusion
Whether it’s via partnerships involving significant inventions or impacts on daily operations, AI is likely to significantly impact future businesses, given the current trajectory of events.
Generative AI has the potential to revolutionize several industries, including the medical field, automate content development and duplication, and more. This encompasses not just the arts and healthcare but also advertising and robotics. This may be problematic to some, but they must be open-minded and remember that generative models are meaningless without human input.
Looking to turn the tables of AI to your advantage, Parangat Technologies is the way to go. We have a team of technology experts with a deep understanding of AI and are set to bring them into action to give wings to your brand. Contact us today to bring next level solutions to life, setting the stage of empowerment and growth.
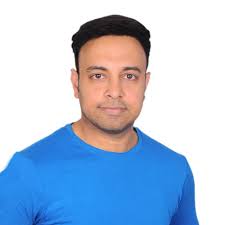
With roll up sleeves, dive in and get the job done approach, it was in the year 2010 when Sahil started Parangat Technologies. Emphasizing a healthy work culture and technology-driven company, he has successfully created a workplace where people love to work and live. He is a software engineer and a passionate blockchain enthusiast.