CAMBRIDGE, MASS. — AI featured heavily in presentations earlier this week at the conference EmTech MIT, hosted by MIT Technology Review. As pressure to experiment with generative AI mounts, organizations are confronting a range of challenges, from practical issues such as domain-specific accuracy to security and privacy risks.
Generative AI is already finding diverse applications in enterprise settings. Hillery Hunter, CTO and general manager of innovation at IBM Infrastructure, noted in her talk “Data Implications in a Generative AI World” that early use cases include supply chain, customer support, contracts and legal. “Enterprises are starting to understand that AI’s going to hit them in a number of different ways,” Hunter said.
As organizations explore the wide range of use cases for generative AI, interest in open source options has increased. Whereas proprietary licensed access to popular large language models such as GPT-4 can be restrictive and costly over the long term, open source alternatives are often cheaper and more customizable.
Taking advantage of open source options can thus enable companies to reduce reliance on vendors and build specialized internal tools tailored to specific tasks and workflows. But responsibly integrating open source generative AI into the enterprise will require rigorously evaluating risks around security, ethics and technical capabilities.
Evaluating open source vs. proprietary generative AI
In her presentation “Open-Sourcing Generative AI,” Alison Smith, director of generative AI at Booz Allen Hamilton, outlined the opportunities and risks of open source generative AI.
Smith highlighted the historical link between the open source community and AI breakthroughs, with major machine learning advancements linked to open source initiatives such as TensorFlow and PyTorch. In the generative AI era, however, the most performant LLMs to date have been closed.
But generative AI is a fast-moving field, and the balance could shift in the future, particularly for niche applications. Open source’s low cost, flexibility and transparency make it attractive to organizations looking to fine-tune models for niche applications and vet source code for security vulnerabilities.
“There’s always been a debate about open source versus closed and proprietary,” Smith said in an interview with TechTarget Editorial. “And I think that has only been amplified because of the power of these generative AI models.”
Although consumer-facing generative models such as ChatGPT are still mostly proprietary, Smith sees open source gaining traction for narrower applications where it can provide specialization, transparency and cost advantages. “It’s really hard to make these blanket statements around open source for everything or not at all,” she said.
Instead, deciding whether to use proprietary versus open source generative AI will likely involve examining the details of specific use cases. Smith mentioned the possibility of an ecosystem emerging of smaller open source models tailored to specific tasks, while consumer applications such as ChatGPT remain largely proprietary.
“I don’t think we expect to see one versus the other at this really broad, umbrella generative AI level,” Smith said. “ChatGPT is so great because you can ask it whatever you want and do all kinds of wild tasks. But do you really need that for specific enterprise-level use cases? Probably not.”
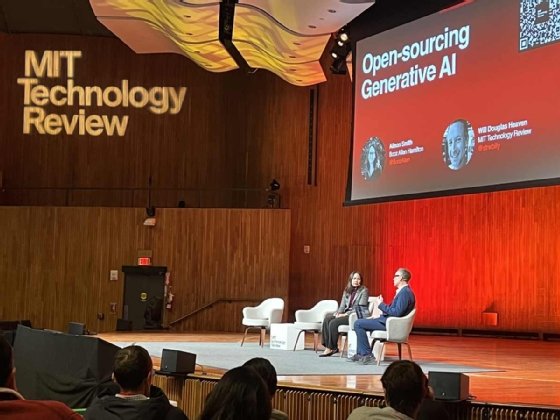
The difficulty of developing open source generative AI
Despite its benefits, building open source generative AI is likely to prove challenging. Compared with other types of software and even other areas of machine learning, generative AI requires much more extensive infrastructure and data resources, as well as specialized talent to build and operate models.
These factors all present potential barriers to building effective open source generative AI. The financial and compute resources required to train useful, high-performing models can be challenging to acquire even for well-funded enterprises, let alone model developers working in an open source context. These funding challenges, although heightened in AI development, reflect longstanding, widespread problems in open source.
“Even with open source software, no matter how popular a framework or a library — even Python as a programming language — it really is a thankless job for all the people who maintain it,” Smith said. “And it’s difficult to commercialize.”
Smith mentioned freemium models that offer free access to basic capabilities while charging for certain features or services, such as security or consulting, as one potential funding approach. Other common models include crowdfunding, support from not-for-profit organizations and funding from big tech companies. The latter, however, can raise tricky issues around influence over the development of open source models and software.
“What’s so great about the open source community is that the contributions come from a huge, diverse pool of people,” Smith said. “And whenever you concentrate funding, you’re going to probably overrepresent that concentrated funding source’s incentives.”
Anticipating and managing enterprise generative AI risk
Effectively deploying generative AI in the enterprise — especially in contexts that make the best use of the technology by fine-tuning on domain- and organization-specific data — will require carefully balancing risk management with innovation.
Although many organizations feel pressure to rapidly implement generative AI initiatives, deploying powerful probabilistic models requires forethought, especially for sensitive applications. A model that deals with information pertaining to healthcare or national security, for example, involves greater risk than a customer service chatbot.
For enterprise applications of generative AI such as internal question-answering systems, Smith emphasized the need for controls on user access and permissions. Just as employees can access only certain folders within their organization’s document management system, an internal LLM should only provide information to users authorized to receive it. Implementing proper access controls can ensure users only get responses that are appropriate for their roles.
“It’s always important to start by enumerating possible risks,” Smith said. “[First] you start with how serious it is, whatever action you’re trying to accomplish, and second, how widespread it is — how many people will be impacted.”
Given that anticipating every risk isn’t likely to be possible, planning carefully and developing incident response policies is crucial. “For me, the best practice is to actually start with the security posture first,” Smith said, then move on to questions of effectiveness and user experience.
Hunter noted that IBM’s research has found that planning ahead can significantly reduce the cost associated with a cybersecurity incident. In the 2023 edition of IBM’s annual “Cost of a Data Breach Report,” organizations with high levels of incident response planning and testing saved nearly $1.5 million compared with their low-level counterparts.
“I think we tend to — especially when we’re excited about new technology — get very star-struck by the benefits,” Smith said. “I almost wish that we would have a conversation about the risks first, where you’re detailing … all the things that could go wrong, knowing that there are even more things than that. And then, based on these benefits, does it make sense?”
Lev Craig covers AI and machine learning as the site editor for TechTarget Enterprise AI.