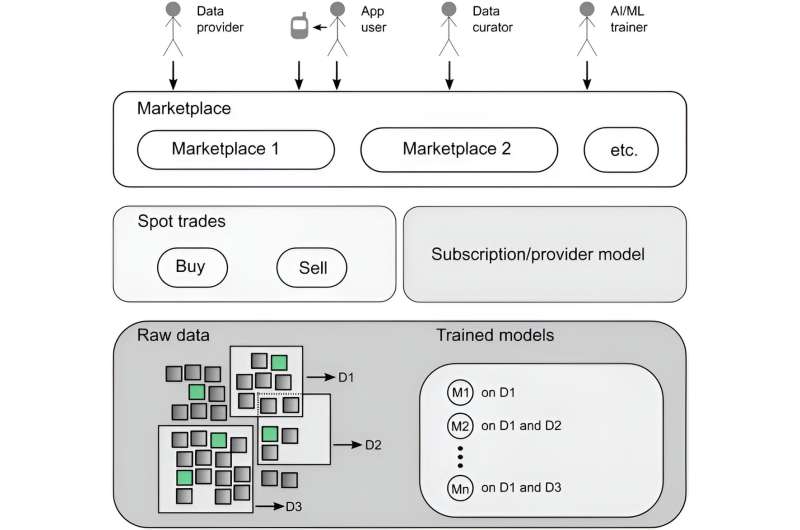
Plant phenotyping which explores the interplay between plant genotypes and their environment, has advanced with automated, high-throughput screening in greenhouses. However, managing the resulting extensive datasets is complex, and sharing such data is restricted due to its costly and technical nature.
Despite existing data management standards like MIAPPE and FAIR, challenges persist in machine learning (ML) applications, primarily due to the need for large volumes of high-quality, labeled data. The reluctance of stakeholders to share data, driven by property rights concerns, hinders progress.
In July 2023, Plant Phenomics published a perspective article titled “Can Distributed Ledgers Help to Overcome the Need of Labeled Data for Agricultural Machine Learning Tasks?.” This paper promotes a collaborative approach in data handling and AI model training for plant phenotyping and suggests using distributed ledger technology with smart contracts to overcome current limitations.
The current system faces significant challenges, including non-standardized data acquisition and processing, risks of eroding property claims, potential data misuse, and a lack of data harmonization. Although standards like MIAPPE or ISA-TAB can address some of these issues, concerns about data privacy, misuse, and the absence of sufficient incentives for sharing persist.
The proposed ecosystem aims to resolve these by leveraging distributed ledger technology, known for secure and accountable data sharing, along with incentive structures to encourage participation. This distributed ledger-based system not only offers financial benefits but also fosters collaboration and electronic governance within a dynamic, interoperable, and decentralized network. It envisions a collaborative environment where stakeholders like data users, providers, curators, and AI/ML model creators actively participate.
To avoid monopolies and encourage diversity, the proposal suggests multiple interoperable marketplaces, allowing stakeholders to choose while accessing the entire ecosystem. This approach also includes e-governance mechanisms, reducing dependence on intermediaries and creating a neutral platform for all participants. However, challenges such as privacy concerns, integration with legacy systems, legal compliance, and the need for a settlement mechanism between tokens and fiat currencies are acknowledged.
In the context of agriculture, this ecosystem has the potential to revolutionize data collection and utilization, particularly in disease prediction and yield forecasting. Farmers could play dual roles as both data providers and consumers, contributing to and benefiting from more accurate ML models. This would enhance the reliability of agricultural forecasts and decision-making, leading to improved crop management and productivity.
In conclusion, this approach presents a comprehensive solution to current data sharing challenges in plant phenotyping. By connecting diverse datasets with metadata and incentivizing stakeholders, it aims to enhance the accuracy and utility of ML models in agriculture, potentially transforming modern farming practices.
More information:
Stefan Paulus et al, Can Distributed Ledgers Help to Overcome the Need of Labeled Data for Agricultural Machine Learning Tasks?, Plant Phenomics (2023). DOI: 10.34133/plantphenomics.0070
Provided by
NanJing Agricultural University
Citation:
Sharing agricultural data: Distributed ledger technology for enhanced machine learning in plant phenotyping (2023, December 20)
retrieved 20 December 2023
from https://phys.org/news/2023-12-agricultural-ledger-technology-machine-phenotyping.html
This document is subject to copyright. Apart from any fair dealing for the purpose of private study or research, no
part may be reproduced without the written permission. The content is provided for information purposes only.