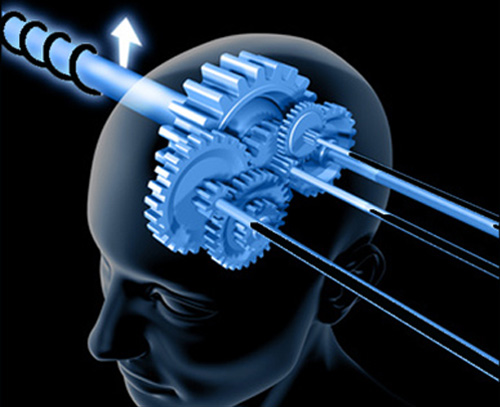
A new study suggests that predicting clinical outcomes based on machine learning models can be slightly better than chance when extended beyond the trials they are developed from.
Researchers found that a machine learning model designed to predict which patients with schizophrenia would benefit from a particular antipsychotic medicine was unable to generalize to other, independent trials.
The results stress the need for robust revalidation to avoid overly optimistic results from machine learning models that may be unable to generalize to wider clinical contexts, said Frederike Petzschner, PhD, from Brown University, in a Perspective accompanying the findings in the journal Science.
“The findings not only highlight the necessity for more stringent methodological standards for machine learning approaches but also require reexamination of the practical challenges that precision medicine is facing,” she added.
A fundamental issue in medicine is that, despite similar treatments, some patients get better while others show no improvement.
Machine learning has been promoted as a tool to improve precision medicine by rooting through large quantities of complex data to identify the genetic, sociodemographic and biological markers that predict the correct treatment for a particular individual at a particular time.
Researchers typically split participants in a particular study into two or more random groups, building a model using one set and then testing the predictions on the other.
However, these models are not usually tested on new patients in a different context as data can be scarce and expensive.
Adam Chekroud, PhD, from Yale School of Medicine, and colleagues therefore examined the generalizability of clinical prediction models across multiple five, international randomized clinical for antipsychotic treatments in patients with schizophrenia.
For this, they used the Yale Open Data Access (YODA) Project, a data-sharing archive of over 246 clinical trials from all medical fields.
All patients had a current DSM-IV diagnosis of schizophrenia at the start of the trial and were randomly assigned to receive an antipsychotic medication or placebo.
The trials used the same Positive and Negative Syndrome Scale (PANSS) to measure outcomes, and all included a four-week timepoint to measure outcome, with similar data collected about the patients at baseline.
The researchers applied machine learning methods using baseline data to predict whether patients would achieve clinically significant improvements in symptoms from four weeks of antipsychotic treatment.
They then examined applicability of the machine learning models across four distinct scenarios to gain insights into their generalizability.
When the models were tested in the sample on which they were developed, they routinely produced strong predictions, but this was tempered by cross-validation.
Importantly, even those models that performed well in cross-validation operated little better than chance when predicting outside of the sample in which they were developed.
The researchers give three reasons predictive models may not generalize across trials. Firstly, the patient groups may be too different across the trials, with patients in different disease stages of schizophrenia included in the same category in the clinical trials.
The trials may also not have collected the type or volume of data needed to make good predictions.
Finally, patient outcomes may be too dependent on context, with the trials having subtly important differences in recruiting procedures, inclusion criteria, or treatment protocols.
“The present study offers an underwhelming but realistic picture of our current ability to develop truly useful predictive models for schizophrenia treatment outcomes,” the researchers report.
“Models that performed with excellent accuracy in one sample routinely failed to generalize to unseen patients.
“These findings suggest that approximations based on a single data set are a fundamentally limited insight into future performance and represent a potential concern for prediction models throughout medicine.”