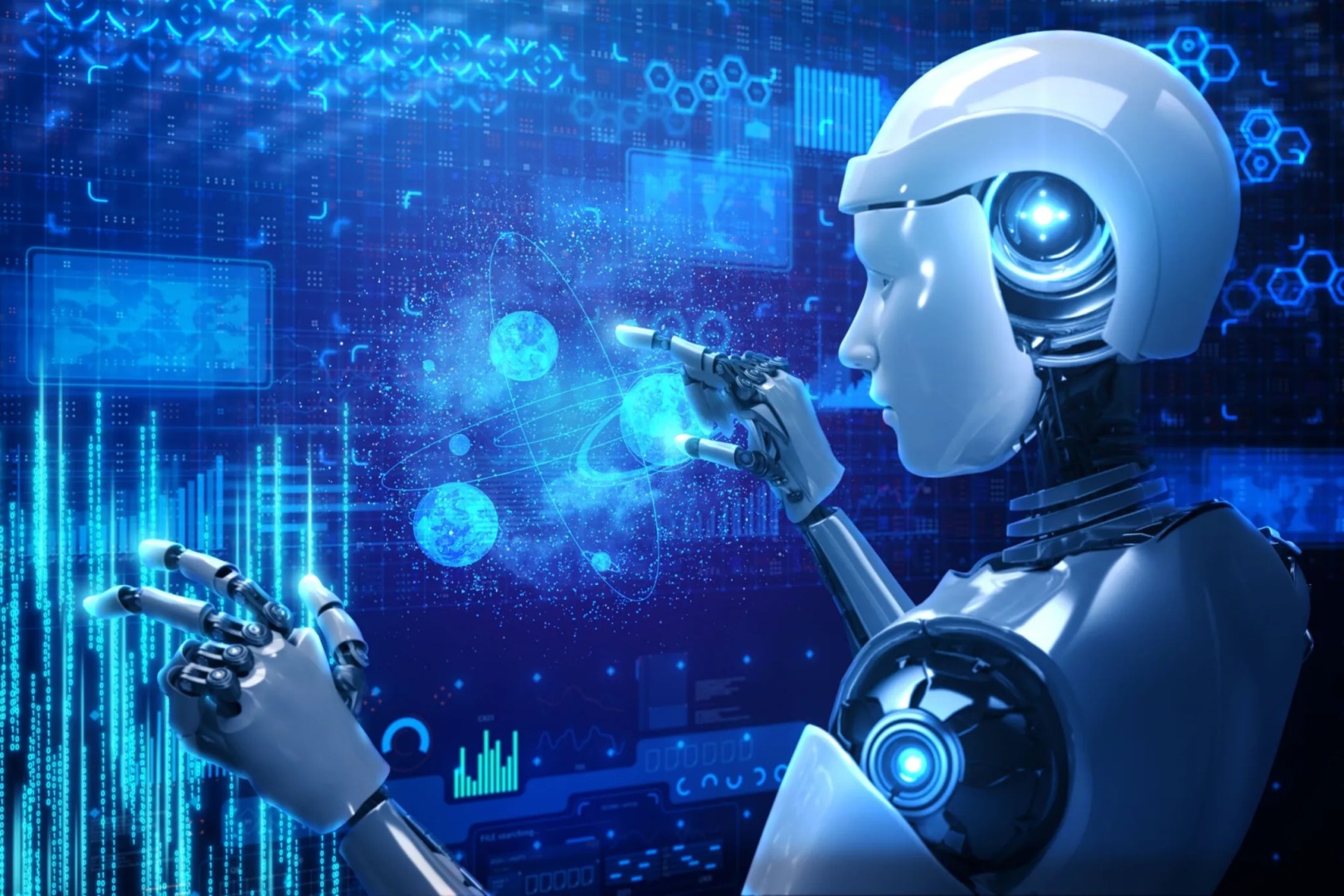
The Generative AI bubble is on the verge of bursting in 2024 due to a convergence of legal challenges, technological limitations, and the need for a fundamental shift in conceptual frameworks.
Programs like ChatGPT and Bard, celebrated for their potential to transform various facets of human life, face scrutiny as the anticipated disruptions seem elusive.
As the tech industry seeks to overcome numerous challenges, a recalibration is necessary to move from overhype to practical efficacy, ensuring the sustainable evolution of Generative AI with ethical considerations at its core.
The Discrepancy Between Promise and Reality of Generative AI
While the potential of Generative AI (GenAI) to synthesize vast amounts of data and usher in breakthroughs in science, economics, and social services was touted, the observed outcomes have been characterized by commercial overhype.
The prevalence of deep fakes, disinformation, and superficial interactions has raised questions about the actual impact of Generative AI on these domains.
A Paradigm Shift is Needed for Generative AI
As we look forward to 2024, there’s a compelling argument for a paradigm shift in the conceptual foundations of GenAI.
The defective conceptual assumption of imitating human intelligence, encompassing the body, brain, behavior, and business, has hindered the true potential of Generative AI. The call for a transition from imitating to understanding and leveraging “world knowledge” becomes imperative.
Generative AI‘s reliance on rote memory and pattern matching, devoid of real intelligence, learning, analogy, or deduction, has limited its capabilities.
The call for a move beyond mathematical induction and statistical inference is paramount for genuine advancements.
Generative AI systems like DALL-E and ChatGPT, trained on unlicensed materials, face a critical challenge in provenancing original source materials. The potential for lawsuits, with settlements ranging from millions to billions of dollars, poses a threat to the sustainability of GenAI.
The defective business model, coupled with the legal expenses involved in lawsuits, creates an untenable situation for big tech companies like Microsoft or Google. The pricing model of the ChatGPT API, despite prospective token profits, raises concerns about the financial viability of the GenAI landscape.
The shift from word embeddings to world embeddings introduces the concept of Trans-AI, wherein Entity Embeddings (EEs) play a crucial role. EEs, representing categorical or ordinal variables, enhance the AI’s ability to interact effectively with the world, moving beyond token-centric representations.
World embeddings enable AI/ML/LLM models to interact with the world more efficiently, understanding semantic and syntactic relationships between tokens. The integration of world knowledge becomes instrumental in generating intelligible content.
Generative AI is Not Perfect
The promises of GenAI have faced a reality check, prompting a reevaluation of its fundamental concepts and business strategies. As we enter 2024, the call for a paradigm shift towards world embeddings and a deeper understanding of the world’s complexities emerges as a crucial step for the sustainable evolution of Generative AI. The journey from overhype to practical efficacy requires a recalibration that embraces genuine innovation and ethical considerations.
While generative AI has made remarkable strides, it is important to acknowledge that it is not perfect. Despite its capabilities, there are inherent limitations and challenges that characterize this technology. Some key aspects to consider include:
1. Bias and Fairness Issues
Generative AI models can inadvertently perpetuate biases present in their training data. If the training data reflects societal biases, the AI may produce biased or unfair outcomes, raising ethical concerns.
2. Lack of Common Sense
Generative AI may struggle with common-sense reasoning, leading to outputs that lack context or coherence. This limitation can affect the practical applicability of the technology in complex real-world scenarios.
3. Weak Contextual Understanding
Understanding context remains a challenge for generative AI. The technology may generate content that is contextually inappropriate or misinterpret nuanced information, impacting the accuracy of its outputs.
4. Ethical Problems
The ethical use of generative AI poses challenges, especially in cases where the technology can be exploited to create deepfakes or misleading content. Striking a balance between innovation and responsible use is an ongoing concern.
5. Dependence on Training Data
The quality and representativeness of training data significantly influence generative AI’s performance. Inadequate or biased training data can lead to suboptimal outcomes and limit the model’s ability to generalize across diverse scenarios.
6. Lack of Explainability
Many generative AI models operate as black boxes, making it challenging to understand the rationale behind their outputs. Explainability issues hinder transparency and may pose challenges in certain regulated or safety-critical domains.
7. Vulnerability to Adversarial Attacks
Generative AI models can be susceptible to adversarial attacks, where malicious inputs are designed to mislead the model. Safeguarding against such attacks requires ongoing research and development in security measures.
8. Overfitting to Training Data
Generative AI models may overfit to the specific patterns in their training data, limiting their ability to adapt to new or unseen scenarios. This overfitting can result in outputs that closely mimic the training data but may lack generalization.
Recognizing these imperfections is crucial for responsible deployment and management of generative AI technologies. Ongoing research and development efforts are essential to address these challenges and enhance the robustness, fairness, and ethical use of generative AI in diverse applications.